Substance-related disorders (SRDs) are of two types: substance use disorders and substance-induced disorders.1 SRDs are one of the most serious psychosocial problems in all societies,2 and are a risk factor for suicide3 and conditions such as AIDS.4 Additionally, SRDs have negative economic and non-economic impacts on patients, their families, and society as a whole. It is considered one of the major public health problems in all countries regardless of their socioeconomic status.
Based on the Global Burden of Disease (GBD) study, substance abuse was responsible for 20 million disability-adjusted life years at the global level in 2010.5 It has also been estimated that more than 2% of total burden of diseases at the global level are associated with SRDs.6 Similar to other developing countries, the burden of substance abuse is high in Iran and has been identified as the third leading cause of disease burden among males.7
Iran, considering its geographic location, is a transit route to substances from countries such as Afghanistan and Pakistan to countries in Europe. Its location is one of the main contributors to the higher prevalence of substance abuse.6,8 In addition, the higher prevalence of substance abuse and easier access to substances impact harm reduction outcomes among substance abuse patients. Over the past decades, several preventive programs have been launched to reduce the prevalence of SRDs in Iran. Harm reduction programs in 1994 and methadone maintenance therapy (MMT) in 1999 are two examples of such programs.9 While the main goal of all these programs is harm reduction of substance abuse, there are some concerns regarding their success and continued abstinence. In previous studies, factors affecting the success of harm reduction included psychological, socioeconomic, and environmental factors, plus the quality of health care and family support.10–13
Although there are several reports regarding relapse among patients with substance abuse, none of them used the decision tree (DT). The DT is an easy to understand tool for both health policymakers and health care providers. Having useful information about the main determinants of harm reduction outcomes among SRDs patients is an important step toward a reduction in relapse rates, better patient management, and increased quality of life. Therefore, we sought to determine the related factors affecting the prevalence of relapse in a large sample of self-referred patients to MMT centers in Kermanshah, western of Iran, using the DT analysis and logistic regression model.
Methods
This cross-sectional study was carried out in the Kermanshah province. Kermanshah is located in the western region of Iran and consists of 14 districts. The Kurdistan province borders it to the north, Hamadan and Lorestan provinces to the east, and Ilam province to the south. It is also bordered by Iraq to the west. The total population within the province was estimated to be approximately two million in 2015. The province has 95 MMT centers for the provision of services to patients with SRDs.
For this study, we included the available data in MMT centers in Kermanshah province in 2015. According to the guideline provided by the Ministry of Health and Medical Education, the same health care services are available for all patients in all MMT centers. We randomly selected 45 out of 95 of MMT centers and included all of their patients. All required information for registration of patients was collected through interviews with participants by full-time psychologists in the centers. Sociodemographic data such as age, sex, marital status (single, married, divorced), education level (illiterate or number of schooling years), and other relevant information including age at first substance use, money spent for buying substance per day, history of substance abuse (yes, no), type of substance (hallucinogen substance, stimulant substance, opium), frequency of substance use (every day, every other day, once a week), and frequency of methadone therapy in MMT centers (every day, every other day, once a week) were collected through interviews.
We included all patients who received methadone for at least one year. Those who refused to provide a urine opium test at specified times were excluded. It should be mentioned that in MMT centers, patients need to give urine samples monthly by request from a physician or psychologist. If the test result was positive, this was regarded as a relapse. Given that some persons may receive methadone by several centers, we used the four digits of clients’ ID cards to identify and exclude duplicated cases.
We defined substance abuse using the criteria provided by the World Health Organization.14,15 Accordingly, substance abuse is the state of periodic or chronic intoxication detrimental to the individual and society, produced by the repeated consumption of a substance (natural or synthetic).15,16 Substance dependence was measured by using the Diagnostic and Statistical Manual of Mental Disorders version 5 (DSM-V).
DT analysis was used to explore data to select the best independent determinants with the best cut-off point. Compared to regression models, DT analysis can automatically take into account interactions between variables. The Gini index was calculated to determine the cut-off point of nodes. Also, the Classification and Regression Trees algorithm was used to create the DT. This algorithm creates two branches in which the branches have maximum homogeneity within each node and maximum heterogeneity between them. To avoid an over-fitted tree, the minimum sample size for the parent node was 100 patients. Furthermore, the final model was pruned and complexity parameters were plotted against the number of terminal nodes.
We used the Gini index to split the root node. For the confidence, the relapse rate (76.6%) was used to create the DT. The data was divided into two parts, training sample (80.0%) and test sample (20.0%). Firstly, using the training sample, the conceptual model was created; then using test sample, the final model created. Finally, model accuracy was calculated using specificity and sensitivity. Nearly 1.0% of data was missing, which was excluded from analyses. Also, the determinants of relapse rate were examined by univariate and multiple logit regression models. All analyses were performed using SPSS Statistics (IBM Corp. Released 2013. IBM SPSS Statistics for Windows, Version 22.0. Armonk, NY: IBM Corp.) with a significance level set at 0.05. The study was conducted according to the Helsinki Declaration and was approved by the Ethical Committee of Kermanshah University of Medical Sciences.
Results
A total of 4175 self-referred patients to MMT centers participated in the study of which 96.7% (4037) were male. The average of age of males and females were 40.0±11.3 and 40.0±12.6 years, respectively (p = 0.690). The mean of age at first substance use was 24.1±8.2 years in males and 29.7±10.5 years in females (p > 0.001). The overall relapse rate was 76.6% with no significant differences between men and women (76.6% vs. 75.4%, respectively).
Univariate and multiple regression models revealed that sex, marital status, and years of schooling were not significantly associated with the relapse rate (p > 0.050) [Table 1]. While in the univariate model, the type of substance use and frequency of substance use before methadone therapy were associated with relapse, but there was no significant association between these variables and relapse in the multiple regression model. In addition, age at first substance use, the total budget for buying substance per day (US$), history for quitting without methadone therapy, and frequency of substance use before quitting were associated with relapse in the multiple regression model (p > 0.050).
Table 1: Factors related to relapse in self-referred addicts to methadone maintenance therapy centers by univariate and multiple regression models.
Sex |
|
|
|
Male |
3091/4037 (76.6) |
1 |
- |
Female |
104/138 (75.4) |
0.92 (0.62–1.37) |
- |
Marital status |
|
|
|
Single |
652/870(74.9) |
1 |
- |
Married |
2346/3056 (76.8) |
1.14 (0.62–1.37) |
- |
Divorced |
181/245 (73.9) |
0.94 (0.62–1.37) |
- |
Education, years |
|
|
|
Illiterate |
167/206 (81.1) |
1 |
- |
≤ 5 |
635/759 (83.7) |
1.19 (0.80–1.78) |
- |
6–9 |
937/1184 (79.1) |
0.88 (0.60–1.28) |
- |
10–12 |
1149/1559 (73.7) |
0.65 (0.45–0.94) |
- |
≥ 13 |
310/463 (66.9) |
0.47 (0.31–0.71) |
- |
Age at first drug use, years |
|
|
|
< 15 |
222/311 (71.4) |
1 |
1 |
15–19 |
1090/1467 (74.3) |
1.24 (0.93–1.65) |
1.13 (0.85–1.51) |
20–24 |
738/951 (77.6) |
1.65 (1.23–2.24) |
1.38 (1.01–1.87) |
25–29 |
542/670 (80.9) |
1.79 (1.30–2.46) |
1.76 (1.27–2.45) |
30–39 |
397/526 (75.5) |
1.41 (1.01–1.97) |
1.31 (0.94–1.82) |
≥ 40 |
211/250 (84.4) |
2.45 (1.52–3.90) |
2.32 (1.49–3.59) |
Total budget buying substance per day, US$ |
|
|
|
< 2 |
962/1337 (72.0) |
1 |
1 |
2–4.9 |
571/812 (70.3) |
0.92 (0.76–1.11) |
0.92 (0.75–1.12) |
5–9.9 |
361/465 (77.6) |
1.35 (1.05–1.73) |
1.38 (1.06–1.79) |
10–14.9 |
565/699 (80.8) |
1.64 (1.31–2.05) |
1.63 (1.30–2.07) |
≥ 15 |
741/862 (86.0) |
2.38 (190–2.99) |
1.41 (1.91–3.07) |
Frequency of methadone therapy in MMT centers |
|
|
|
Each day |
1420/1988 (71.4) |
1 |
- |
Every other day |
501/590 (84.9) |
2.25 (1.76–2.88) |
- |
Once a week |
1279/1597(80.1) |
1.68 (1.37–1.88) |
- |
History for quitting without treatment |
|
|
|
No |
261/656 (39.8) |
1 |
1 |
Yes |
2939/3300 (89.1) |
3.27 (2.43–4.40) |
2.14 (1.69–2.71) |
Frequency of substance use before abstinence |
|
|
|
Every day |
1014/1293 (78.4) |
1 |
1 |
Every other day |
299/342 (87.4) |
1.91 (1.35–2.70) |
1.90 (1.30–2.78) |
Once a week |
1887/2540 (74.2) |
0.79 (0.67–0.93) |
0.76 (0.64–0.91) |
Types of substance |
|
|
|
More than one drugs |
309/362 (85.4) |
1 |
- |
Hallucinogen |
329/450 (73.1) |
0.46 (0.32–0.66) |
- |
Stimulant drugs |
255/331 (77.0) |
0.57 (0.39–0.84) |
- |
Opium related drugs |
2090/2731 (76.5) |
0.55 (0.41–0.75) |
- |
Substance use disorder |
|
|
|
No |
230/347 (66.3) |
1 |
- |
OR: odds ratio; CI: confidence interval.
All explanatory variables [Table 1] were used as quantitative variables to create the DT and the Gini index to calculate all cut-off points. The DT resulted in a five-level model. The sensitivity of training and testing groups were 74.0% and 72.0%, respectively. Also, the specificity of these groups were 66.0% and 65.0%, respectively. The accuracy rate of training and testing models were 70.0% and 68.0%, respectively.
The first level of the DT was defined by the average daily budget for buying substances. The relapse rate for those who spent more than US$5 per day buying substance with SRDs for more than one substance was 91.7% compared to 54.5% in those addicted to only one substance. In addition, no history of methadone treatment in those who received methadone once or twice a week decreased the risk of subsequent relapse (64.8% vs. 88.1%). Among those who spent less than US$5 per day buying substances and those who more frequently consumed substances (seven times or more per month), being on daily methadone therapy increased the risk of relapse (88.0% vs. 70.7%). If the age of first substance use was less than 19.5 years old, the rate of relapse reach was 76.5% versus 62.9% in those who started to use substances at 19.5 years of age or older [Figure 1].
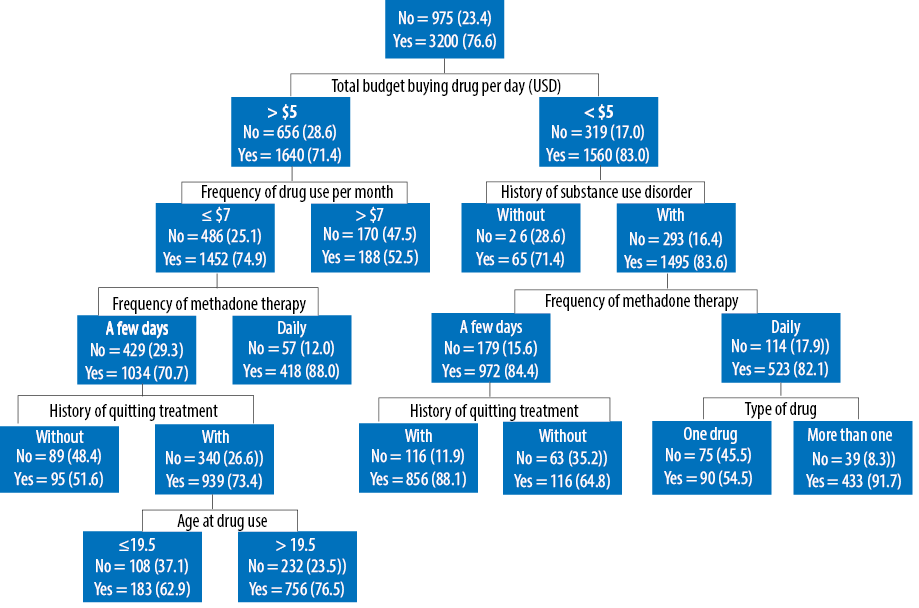
Figure 1: Decision tree analysis of factors related to relapse in patients with substance-related disorders receiving methadone maintenance therapy.
Discussion
This is the first attempt to evaluate the factors affecting the relapse rate in people with SRDs using DT analysis and the logistic regression model. Given the different characteristics and frequency of effective factors on harm reduction and treatment among people referred to MMT clinics, developing a prognostic model is a cornerstone to help physicians to make better treatment choices and then to improve treatment outcomes. We found no similar study for comparing the results and validation of the model. It is necessary to examine the validation of the model in future similar studies.
The results of our study revealed that the overall relapse rate is 76.6%, which is different from both national and international studies. For example, studies conducted in different parts of Iran reported relapse rates of 30.4%17 and 72%.18 In the US, about one-third of people relapsed in the first year, and two-thirds relapsed over 10 years after quitting.19 A relapse rate of 91% has also been reported in Dublin, Ireland.20 Another study from San Diego showed that about two-thirds (67%) of adults used substances when they were at a social event.21 Nevertheless, a high relapse rate in the region, a downward trend in the age-adjusted rates of fatal substance overdose in both genders has been reported in Kermanshah province. In fact, harm reduction or treatment for substance addiction has a complex nature, which is associated with social, cultural, environmental, and personal factors. All these factors may lead to differences in relapse after harm reduction and treatment.
Several studies have shown that education level is a predictor of treatment outcome.22–24 In this study, however, education level did not remain in the DT and multiple logistic regression models. We expected to have education as one of the main determinants of relapse in the final model and DT. Educated people have more self-efficacy with better psychosocial and personality characteristics that indirectly affect both the onset of substance use and experiencing successful abstinence.
Patients who expend more than US$5 per day for buying substances had a better treatment outcome. In this group, about half of those patients who used substances less than seven days per month had successful treatment. In our opinion, the higher expenditures for buying substances may reflect the economic status of the patients. In this regard, a survey25 conducted among 111 adolescents to identify the relationship between compulsive buying and risky behaviors reported a significant correlation between uncontrolled buying in college students and substance abuse.
The DT also indicated that among patients who used substances more than seven days per month, having occasional MMT (not daily) has a positive impact on the treatment outcome. In addition, within the group of these subjects, those who had a history of quitting substance without medication had 48.4% success rate compared to 26.6% of those without the history of quitting. In line with our results, a study conducted to determine factors associated with illicit opioid use showed that daily MMT dosages were associated with illicit opioid use.26 Although we did not have any information about the depression status of our patients, the treatment of depressive symptoms may be an important issue to improve treatment outcomes in substance abuse patients.27
Patients aged > 19.5 years at first substance use with no history of substance withdrawal had better treatment success. Similarly, the previous epidemiological studies indicated that a younger age at first substance use was associated with an increased risk of substance abuse disorders and the rate of relapse.28–30 One reason may be the interaction between genetic and other environmental factors, although this causal mechanism is not well known yet.31
The results from another arm of the DT showed that the treatment success rate depends on the variable stratified. For example, patients who spent less than US$5 per day buying substances and had no other SRDs had better treatment outcomes (83.0% vs. 71.4% relapse rate). A positive treatment outcome was observed in individuals with a comorbidity and SRDs (83.6% vs. 71.4%) with daily MMT (82.1% vs. 84.4%) and with single substance use (54.5% vs. 91.7%).
This study has several limitations. We conducted a cross-sectional study design, and the associations should be interpreted cautiously. On the other hand, data from medical records were used and some variables were also collected based on self-reported data, which raises the issue of information bias. In addition, we had no access to the cohort’s psychological functioning, social and family support system, and socioeconomic status. Despite the mentioned limitations, our results can be important and provides additional information for physicians to make better decisions for improving treatment outcomes.
Conclusion
Variables such as substantial expenditures, age at first substance use, history of quitting substance without medication, frequency of substance utilization per month, and frequency of methadone therapy effect on the substance abuse relapse. In addition, the DT based approach revealed a useful approach to show the effect of these factors on the treatment result at different levels.
Disclosure
Authors declared no conflicts of interest. No funding was received for this study.
Acknowledgments
The authors would like to thank the Substance Abuse Prevention Research, Health Institute, Center and Clinical Research Development Unit of Farabi Hospital, University of Medical Sciences, Kermanshah, Iran for their support, cooperation, and assistance throughout the period of study.
references
- 1. Maruf MM, Khan MZ, Jahan N. Pattern of substance use: study in a de-addiction clinic. Oman Med J 2016 Sep;31(5):327-331.
- 2. Juibari TA, Behrouz B, Attaie M, Farnia V, Golshani S, Moradi M, et al. Characteristics and correlates of psychiatric problems in wives of men with substance-related disorders, Kermanshah, Iran. Oman Med J 2018 Nov;33(6):512-519.
- 3. Shakeri J, Farnia V, Abdoli N, Akrami MR, Arman F, Shakeri H. The risk of repetition of attempted suicide among Iranian women with psychiatric disorders as quantified by the suicide behaviors questionnaire. Oman Med J 2015 May;30(3):173-180.
- 4. Khamis F, Al Noamani J, Al Naamani H, Al-Zakwani I. Epidemiological and clinical characteristics of HIV infected patients at a tertiary care hospital in Oman. Oman Med J 2018 Jul;33(4):291-298.
- 5. Degenhardt L, Whiteford HA, Ferrari AJ, Baxter AJ, Charlson FJ, Hall WD, et al. Global burden of disease attributable to illicit drug use and dependence: findings from the Global Burden of Disease Study 2010. Lancet 2013 Nov;382(9904):1564-1574.
- 6. Moazen B, Shokoohi M, Noori A, Rahimzadeh S, Saeedi Moghaddam S, Rezaei F, et al. Burden of drug and alcohol use disorders in Iran: findings from the global burden of disease study 2010. Arch Iran Med 2015 Aug;18(8):480-485.
- 7. Feigin VL, Forouzanfar MH, Krishnamurthi R, Mensah GA, Connor M, Bennett DA, et al; Global Burden of Diseases, Injuries, and Risk Factors Study 2010 (GBD 2010) and the GBD Stroke Experts Group. Global and regional burden of stroke during 1990-2010: findings from the Global Burden of Disease Study 2010. Lancet 2014 Jan;383(9913):245-254.
- 8. Asad AZ, Harris R. The politics and economics of drug production on the Pakistan-Afghanistan border: Ashgate Pub Ltd; 2018.
- 9. Alam-Mehrjerdi Z, Abdollahi M, Higgs P, Dolan K. Drug use treatment and harm reduction programs in Iran: A unique model of health in the most populated Persian Gulf country. Asian J Psychiatr 2015 Aug;16:78-83.
- 10. Brown SA, Vik PW, Creamer VA. Characteristics of relapse following adolescent substance abuse treatment. Addict Behav 1989;14(3):291-300.
- 11. Cohn A, Elmasry H, Niaura R. Facility-level, state, and financial factors associated with changes in the provision of smoking cessation services in US substance abuse treatment facilities: Results from the National Survey of Substance Abuse Treatment Services 2006 to 2012. J Subst Abuse Treat 2017 Jun;77:107-114.
- 12. Kanato M, Leyatikul P. Effectiveness of drug camps in treatment of substance abuse in Thailand. J Community Health 2017 Dec;42(6):1090-1095.
- 13. Lui CK, Sterling SA, Chi FW, Lu Y, Campbell CI. Socioeconomic differences in adolescent substance abuse treatment participation and long-term outcomes. Addict Behav 2017 May;68:45-51.
- 14. Smith SM, Dart RC, Katz NP, Paillard F, Adams EH, Comer SD, et al; Analgesic, Anesthetic, and Addiction Clinical Trials, Translations, Innovations, Opportunities, and Networks (ACTTION) public-private partnership. Classification and definition of misuse, abuse, and related events in clinical trials: ACTTION systematic review and recommendations. Pain 2013 Nov;154(11):2287-2296.
- 15. World Health Organization. The ICD-10 classification of mental and behavioural disorders: clinical descriptions and diagnostic guidelines. Geneva: World Health Organization; 1992 [cited 2019 January]. Available from: https://apps.who.int/iris/handle/10665/37958.
- 16. Eddy NB, Halbach H, Isbell H, Seevers MH. Drug dependence: its significance and characteristics. Psychopharmacol Bull 1966 Jul;3(3):1-12.
- 17. Kassani A, Niazi M, Hassanzadeh J, Menati R. Survival analysis of drug abuse relapse in addiction treatment centers. Int J High Risk Behav Addict 2015 Sep;4(3):e23402.
- 18. Shafiei E, Hoseini AF, Bibak A, Azmal M. High risk situations predicting relapse in self-referred addicts to bushehr province substance abuse treatment centers. Int J High Risk Behav Addict 2014 Jun;3(2):e16381.
- 19. Xie H, McHugo GJ, Fox MB, Drake RE. Substance abuse relapse in a ten-year prospective follow-up of clients with mental and substance use disorders. Psychiatr Serv 2005 Oct;56(10):1282-1287.
- 20. Smyth BP, Barry J, Keenan E, Ducray K. Lapse and relapse following inpatient treatment of opiate dependence. Ir Med J 2010 Jun;103(6):176-179.
- 21. Ramo DE, Brown SA. Classes of substance abuse relapse situations: a comparison of adolescents and adults. Psychol Addict Behav 2008 Sep;22(3):372-379.
- 22. Abdollahi Z, Taghizadeh F, Hamzehgardeshi Z, Bahramzad O. Relationship between addiction relapse and self-efficacy rates in injection drug users referred to Maintenance Therapy Center of Sari, 1391. Glob J Health Sci 2014 Feb;6(3):138-144.
- 23. Kheradmand A, Zamani E, Hedayati N. Exploit the developed countries experiences of primary school education to prevent drug addiction and implications for iran. Addict Health 2009;1(1):44-51.
- 24. Mohammadpoorasl A, Fakhari A, Akbari H, Karimi F, Bostanabad M, Rostami F, et al. Addiction relapse and its predictors: A prospective study. J Addict Res Ther 2012;3(01):122.
- 25. Roberts JA, Tanner JF Jr. Compulsive buying and risky behavior among adolescents. Psychol Rep 2000 Jun;86(3 Pt 1):763-770.
- 26. Li W, Wang Z, Liu Z. Factors associated with illicit opioid use in methadone maintenance treatment clients in 5 Provinces, China. Environ Health Prev Med 2016 Nov;21(6):480-486.
- 27. Hasin D, Liu X, Nunes E, McCloud S, Samet S, Endicott J. Effects of major depression on remission and relapse of substance dependence. Arch Gen Psychiatry 2002 Apr;59(4):375-380.
- 28. Chi FW, Weisner C, Grella CE, Hser YI, Moore C, Mertens J. Does age at first treatment episode make a difference in outcomes over 11 years? J Subst Abuse Treat 2014 Apr;46(4):482-490.
- 29. Pilatti A, Godoy JC, Brussino SA, Pautassi RM. Patterns of substance use among Argentinean adolescents and analysis of the effect of age at first alcohol use on substance use behaviors. Addict Behav 2013 Dec;38(12):2847-2850.
- 30. Richmond-Rakerd LS, Slutske WS, Lynskey MT, Agrawal A, Madden PA, Bucholz KK, et al. Age at first use and later substance use disorder: Shared genetic and environmental pathways for nicotine, alcohol, and cannabis. J Abnorm Psychol 2016 Oct;125(7):946-959.
- 31. Hingson RW, Heeren T, Winter MR. Age at drinking onset and alcohol dependence: age at onset, duration, and severity. Arch Pediatr Adolesc Med 2006 Jul;160(7):739-746.